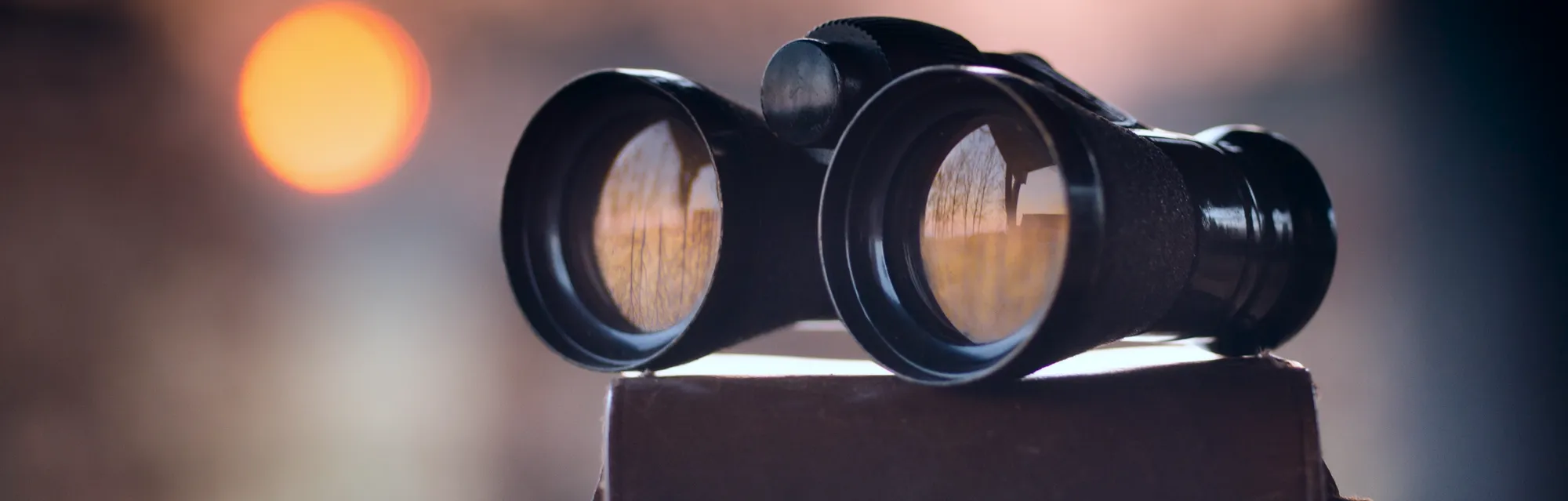
Driving differentiation in e-commerce marketplace search
December 27, 2022 • 11 min read
With tens or even hundreds of thousands of options at their fingertips, shoppers are switching brands at unprecedented rates. While traditional values like price (30%), quality (16%), and delivery costs (15%) are still significant decision-making factors, a report by McKinsey shows that availability (48%) and convenience (34%) are now the strongest drivers of new purchases.
Simply put, shoppers expect to find what they are looking for with ease. If they don’t, they will leave your e-commerce website and buy the item elsewhere. This phenomenon is known as “search abandonment”. According to a recent Google Search Abandonment Survey, in the US alone, bad online search experiences cost retailers over $300 billion annually. Moreover, 77% of consumers who have experienced search difficulties in the past are likely never to visit the website again.
When it comes to online marketplaces in particular, the fight for customers is fierce. How are leading marketplaces like Amazon, eBay and Etsy getting customer experience right? One key factor in delivering exceptional customer experience is through the search bar. In this blog, we outline some of the factors that impede great search and how to overcome them with best-in-class search technology.
Data quality issues hampering retail search experience
Search abandonment has become an industry-wide issue largely because traditional search technologies don’t provide sufficient capabilities for modern-day marketplace e-commerce requirements. Today, people expect instant, precise, relevant and personalized search results closely matching their buying intent. In a world where everyone is used to the Google search bar, this expectation has long ceased to be unreasonable. Yet there are still multiple issues that are holding online marketplaces back from providing a great search experience. We detail some of them below.
Selling online involves offering high-quality goods and supporting buying decisions with superior product data. This includes creating rich product information that enables customers to find, comprehend, and compare items across marketplace vendors, which results in more sales conversions, larger order values, and ongoing brand loyalty.
Helpful, informative, and consistent product descriptions that capture buyers’ attention start with the right attributes, which are the building blocks of the marketplace catalog. They are used as faceting and filtering parameters for navigation, product comparison reports, and promotions. Lack of proper product attribution can make it harder for shoppers to find a product among similar offerings on the marketplace.
In addition to salient features like price, size, and color, product descriptions can also include intangible characteristics like quality, reliability, safety, or aesthetics. This helps retailers create a more compelling and comprehensive story about the product, showing how it meets buyers’ needs and wants, and how they will benefit from getting it.
Managing product information in digital commerce involves multiple parties, including site admins, suppliers, and merchandisers. Retailers apply considerable effort to provide accurate product attributes and attractive descriptions, leveraging standardization and controls in their product information management and merchandising systems.
Having to rely mostly on seller-supplied product data, marketplace operators face great challenges in providing the same level of product data quality across the platform. They often have to deal with the following product data issues:
- Inconsistent product labeling. The ways different sellers label the same product may vary, making it difficult for customers to compare similar products.
- Missing or incomplete product information. In some cases, sellers may provide incomplete product information or even leave out important details such as item dimensions, brand, or material.
- Incorrect product details: Sellers may occasionally provide incorrect product details, such as wrong item dimensions or wrong product images.
- Erratic categorization. Sellers may underutilize or abuse product catalog taxonomy, listing the product under irrelevant categories.
- Excessive Tagging. When sellers add essential product properties as tags instead of using proper attributes, it creates confusion about the meaning of the particular tag, compelling buyers to rely on guesswork rather than faceted search.
- Noisy textual data. Idiomatic expressions, abbreviations, non-standard words, acronyms, internet slang, domain-specific terminology, spelling and punctuation errors create digital noise obscuring essential product information. Moreover, noisy textual data can not be categorized properly by text mining software, leading to lower precision and a lot of false positive results even for simple queries.
- Inaccurate product bundles. Product bundles are a powerful cross-selling tool. However, many sellers tend to misuse this feature, grouping unrelated items in the same product listing, often mixing up their attributes or failing to submit the description that takes into account the entire bundle.
- Untreated duplicates. Marketplaces often have multiple listings of the same item. When these duplicates are not recognized by the system as such, it makes it harder for buyers to navigate product offerings and compare them.
- Search engine manipulations. In attempts to bypass platform algorithms and appear on the 1st search results page, many sellers use SEO hacks to gain customer attention. They often spam product descriptions and/or titles with popular terms, brand names, or other inappropriate keywords that are not part of context-based item characteristics. Other common tricks include hidden text (e.g. “white on white”), tags concealed in metadata, drop-down boxes, and comparisons.
With all those issues, building a high-quality marketplace product search may seem like a pretty challenging task. However, modern information retrieval and machine learning techniques come to the rescue helping to improve both data quality and search results relevance.
Improving marketplace search quality with data enrichment
The marketplace search improvement journey should start with product listing data. Even the most sophisticated search algorithms and machine learning models will produce bizarre results if they are not presented with reliable and comprehensive ground truth.
Data enrichment allows retailers to add value to the purchase decision of their customers. This process is all about finding gaps and inconsistencies in product information and filling them with up-to-date, accurate and relevant inputs. Marketplaces are using a variety of methods to improve data quality:
- Sourcing attributes from approved manufacturer sites, product specifications, images, videos, and customer reviews;
- Encouraging sellers to provide rich product data when creating product listings;
- Leveraging category-specific taxonomies in product onboarding processes to ensure complete and valid product attribute information;
- Attributing product listings using internal workforce or crowdsourcing.
However, restrictive approaches can give rise to a risk of turning sellers away from the platform, while manual attribute verification and review processes do not scale well. Marketplaces need to employ less invasive yet more powerful methods for product data enrichment.
New hope comes from modern Artificial Intelligence (AI) models. They can help to bridge the gap between conflicting requirements for low-friction product onboarding on the seller’s side and structured data that generates rich results for a seamless user search experience.
Product labeling using computer vision and NLP
Product type fidelity is one of the key areas for improvement in marketplace search. The discovery process typically revolves around a specific kind of product a customer is looking for, be it a skirt, power drill, or vase. Therefore, missing product type makes it very hard to find the listing, while flawed labeling leads to irrelevant search results.
Computer vision and NLP models can suggest the proper product type by analyzing all available visual and textual information about the product, including images, titles, and descriptions. When combined, image and text-based solutions reinforce each other achieving a high level of accuracy which simplifies the listing creation process.
AI models go even further. They can perform category-specific attribute extraction, identifying such salient features as dominant color, design, and materials. This enables embedding top labels in the search index, which greatly improves the discoverability of the listing and overall search quality.
Extracting product data from customer reviews
Customer reviews are another valuable source of product data. Running buyers’ feedback through NLP algorithms allows retailers to extract not only key product attributes and subjective characteristics such as quality, reliability, safety, or aesthetics, but also gather and evaluate customer sentiment. Building on this information sellers can create a more compelling, complete story that illustrates what life looks like with the product, why it’s the best match for buyers’ needs and wants, and how they will benefit from getting the desired item.
Leveraging customer search logs in product attribution
Last but not least, the source of additional product information is customer search logs. Diving into buyers’ search history provides valuable insights into search queries which led to viewing or purchasing a particular product. These queries are then analyzed to extract key concepts that can be indexed with the rest of the product data, creating a sort of self-learning product attribution system.
While this approach is quite simple and effective, it is limited to existing product offerings with high user engagement. This issue can be tackled by applying Machine learning (ML) algorithms trained to predict top search queries based on available product information. This way we can identify top engaging queries even for new products based on their visual and attribute similarity with entries we used for model training:
Supercharging marketplace search with AI and ML technologies
Improving and enriching product data is an essential step in achieving high-quality product searches for the marketplace. However, to unlock its full potential, marketplaces should tap into advanced search technologies powered by AI and ML which can leverage refined product data as well as information related to customer search and shopping behavior. The latter includes product views, add-to-cart events, click behavior, and customer purchase history. All this wealth of data is probably already collected for analytics and marketing purposes and can be used to further improve the quality of customer search experience.
Achieving high-quality search matches with query parsing
Before a query can be executed by a search engine, it has to be broken down into several elementary entities. These notions are then mapped and labeled with the role they play, enabling search engines to match a particular term in a particular field. This process is called query parsing.
Semantic query parsing is an invaluable technique in the online commerce environment as it allows users to formulate precise requests that help search engines to retrieve relevant products. This is achieved by analyzing product data and leveraging attributes to build possible interpretations of customer queries. The graph below shows how it works on the example of the search query “m olive shirt dress”:
Improving search ranking with location awareness and business signals
Product data enrichment isn’t the only way marketplaces can use to facilitate customer search experience. They can leverage a lot of additional signals, beyond the simple textual relevance to improve the rankings of the products in search results.
This is especially important for broad queries that don’t provide much context and often refer to the whole product type or even category, e.g. “power drill” or “glass vase”. Given the fact marketplaces usually feature hundreds of power drills and dozens of glass vases, what would be the most appropriate way to rank them in search results?
Marketplaces can capitalize on versatile business signals to improve product ranking in such scenarios. Their toolkit consists of listing freshness, seller reputation, product popularity, and location awareness techniques.
For example, when customers are searching for apparel, garden furniture or camping equipment, personalizing search results to their location can be a real game-changer. Knowing a client’s whereabouts provides retailers with invaluable insights into their mindset, culture, and expectations from a product or service. For example, “coat” and ”shoes” may mean very different products for people in Minnesota and Florida.
Location awareness also allows search engines to acknowledge the language nuances between the UK and the US. Take “black pants” for example. Understanding the difference this query bears in British and American English can help to avoid some bizarre results.
Introducing neural search
Neural search (aka Semantic vector search) is a powerful self-learning product discovery technology that goes beyond keyword matching and superficial textual information in titles, descriptions, and attributes. It captures all available catalog data and customer engagement history, like metadata, images, reviews, ratings, prices and promotions, which is then uniformly encoded and packed into the dense vector representation by the deep learning model.
Instead of searching for keyword matches, neural search technology represents products as semantic vectors. Those vectors, known as embeddings, capture essential product features in such a way that similar products are clustered together in multidimensional vector space. As a result, the whole search dilemma is reduced to two steps:
- encoding the customer query as a vector;
- performing nearest neighbor search in the multidimensional vector space.
The product and query encoders are based on natural language processing (NLP) deep learning models. They can be trained end-to-end using customer behavior data to ensure that queries and products relevant to those queries are embedded close to each other in a multi-dimensional space.
Well-trained neural search models can tackle complex “long-tail” queries which are completely out of reach for traditional keyword-based algorithms, such as out-of-vocabulary, thematic and symptomatic queries. This enables customers to use phrases like“winter is coming” to search for Game of Thrones related apparel or “leaky roof” to find sealants.
Exploring visual content with text-to-image search
By designing their product catalog in a specific manner, marketplaces can engage customers and direct them to the goods they want or need. Since people are visually oriented beings, allowing them to explore the images and image captions can greatly improve search quality.
Modern deep learning models can directly associate text and visual content, representing a conceptual understanding of the search query. This technology known as text-to-image search, enables users to find images (and corresponding products) by textual descriptions.
One example of such technology is the CLIP (Contrastive Language–Image Pre-training) model from Open AI which can be fine-tuned for the specific domain and catalog. This solution comes in handy in categories like home decor, apparel, art, beauty, and furniture which heavily depend on product looks.
Embracing smart autocomplete to maximize search convenience
Autocomplete is the first feature the customer encounters when interacting with the search box. This makes it an important gateway into retail search.
High-quality autocomplete is essential for a smooth customer experience, especially on mobile devices where the smaller screen and keyboard limit the use of more traditional faceted search selectors. With the search-as-you-type feature, users can significantly cut the time to find a particular product in the catalog.
Smart autocomplete tolerates typos, slang, and abbreviations and can suggest relevant completions based catalog contents and popular customer queries. Session intent awareness can further improve the suggestions, creating the impression that the search system “understands” you.
Delivering relevant recommendations
Personalized recommendations are an essential component of e-commerce. This capability allows retailers to delight their customers with relevant suggestions that anticipate their wants and desires with respect to fashion and style. The results that best match the buyer’s initial query are obtained through the combination of the following techniques:
- Visual similarity-based recommendations retrieve a ranked list of catalog items that look similar to the target product, giving customers a better selection and price;
- Visual similarity technology resolves product matching issues by automatically finding and grouping the same products, which enables customers to compare several offerings. In addition, it can detect missing attributes by analyzing product data of identical items provided by different sellers;
- Supercharging traditional collaborative filtering with deep learning can reveal up to 80% more product catalog similarities based on customers viewing and buying behavior;
- Modern session-based recommenders powered by deep learning can accurately capture customer shopping intent and provide relevant recommendations even for extensive catalogs typical for marketplaces;
- Personalization algorithms combine the “wisdom of the crowd” with implicit customer preferences captured by the deep learning model to deliver the best recommendations.
Summary
Online marketplaces can benefit immensely from modern search and AI solutions readily available to make customers’ shopping journeys intuitive, smooth and highly personalized. These technologies significantly improve product data quality and fidelity, taking product discovery and faceted navigation to a whole new level. Employing state-of-the-art techniques powered by NLP and deep learning can enhance the quality of search even further despite all the noisy data typically plaguing the marketplace.
Implementing smart search solutions can distinguish your marketplace from other platforms and create an impression of an intelligent system that fully understands the customer. And we would be more than happy to help you with that. Don’t hesitate to reach out!