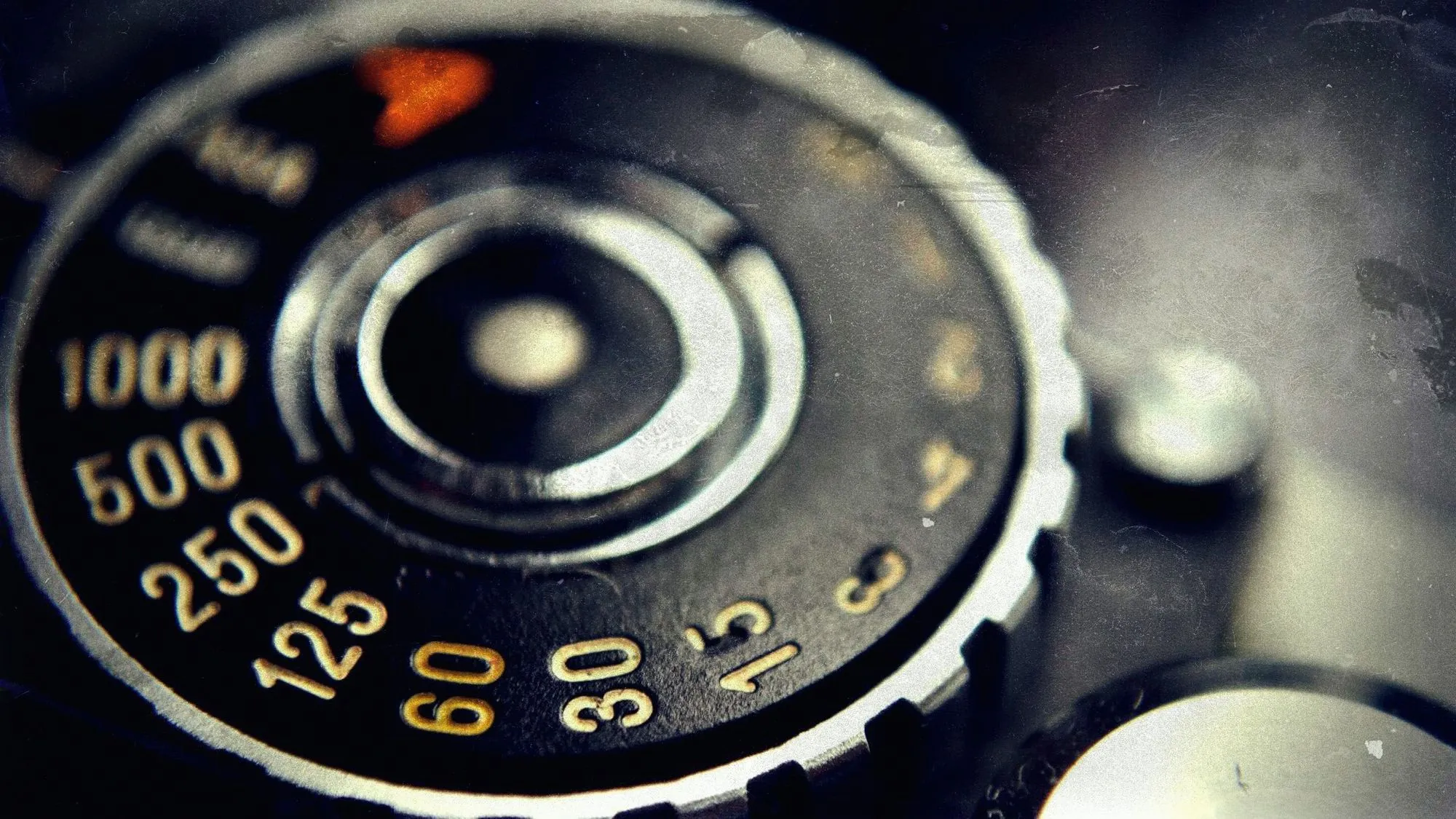
10 ways computer vision is transforming digital retail
October 17, 2019 • 7 min read
- Visual search helps retailers improve customer experience
- Product recommendations create upsell opportunities
- Customer service automates question answering
- Augmented reality (AR) - try before you buy
- Magic mirrors for on-premise fitting rooms
- Categorizing automation improves UX and saves time
- Product attributes are important for accurate searches
- Warehouse automation improves efficiency
- Inventory management controls cost
- Delivery innovations
- Conclusion
Computer vision has become essential to remaining competitive in the digital retail space because it offers innovative customer experiences and process automation that delights customers and cut costs.
Customers demand the convenience of features such as visual search or automated question answering and retailers benefit from the increased operational efficiency of features, such as warehouse automation or more effective product discovery.
In this blog post, we showcase the most interesting computer vision use cases, both present, and future, which have transformed e-commerce.
Listed below are some of the most visible effects of computer vision technology.
Visual search helps retailers improve customer experience
Visual search is one of the most popular ways deep learning technology is utilized to benefit the customer experience and alleviate the limitations of text-based product discovery.
Some search queries are difficult to describe with words and are better suited to a more visual search experience. A project Grid Dynamics completed for Art.com illustrates the advantages of visual search over text in helping customers search for the artwork of a similar style rather than content.
This case study explains how deep learning vectorization models can extract the hard to describe “latent features” of a painting’s artistic style and then provide search results based on products with a similar style.
For example, imagine that you are interested in a painting in the style of old Dutch masters — depicting a bowl of peaches. You are looking for some more pieces of art in the same style.
Let’s compare text and visual search results:
The text search failed your expectations, while the visual search is spot on.
In another example, where shoppers may be interested in seeing a variety of similar outfits, Macy’s.com offers a “see more like this” feature.
This feature enables customers to find visually similar options that are ranked to describe latent features not available in the faceted navigation.
Visual search technology has been very well received by online shoppers and is redefining the online shopping experience.
Given that modern customers expect the search experience to be as convenient and easy as possible, visual search experiences are becoming a requirement for digital retailers that want to maintain their competitive edge.
Product recommendations create upsell opportunities
Retailers like the opportunity to upsell their customers, and customers like the convenience of having relevant products presented during their shopping, especially when there is a discount.
The issue has always been figuring out what customers want.
Collaborative filtering algorithms can look at a large data set of e-commerce activities and figure out the latent features that guide successful recommendations. Even for large catalogs without data on every product, it’s possible to use deep learning to provide quality behavior-driven recommendations relevant to users that create opportunities to upsell.
Customer service automates question answering
In the customer service department, computer vision can take the place of a well-informed salesperson. For example, a customer may ask, “What goes well with this shirt?” or “What style of shoe is this?”
Computer vision is used to identify the object, while artificial intelligence processes the question using Natural Language Processing (NLP).
In an earlier blog, The next big thing in customer service, a deep learning question-answering system, we explore advances in deep learning that will enable automated customer service and potentially automate an essential and expensive aspect of the buying process.
Augmented reality (AR) – try before you buy
Augmented reality combines aspects of the real world, such as backgrounds, with computer-generated content, such as a product.
For example, to see how a piece of furniture or art looks in your house, AR places a computer-generated version into a real image of the room.
Computer vision is required to precisely position the digitized object and rotate it properly to make it appear authentic. Multiple sensory modalities may be altered beyond visual, such as auditory or haptic.
Customers enjoy these immersive experiences which make it easier to buy without seeing a product in person. Retailers should push impressive new features like AR to wow their customers and boost online revenue.
Magic mirrors for on-premise fitting rooms
Magic Mirrors are not a category of online shopping; I decided to include it due to its wow factor.
Magic mirrors are used for on-premise shopping, replacing traditional mirrors. Combining the functionality of a mirror, a monitor, and a computer opens up many possibilities.
The user can see themselves both front and back, view both real-time and recorded views, and use augmented reality to “try on” different outfits virtually. The user can then use online features to complete a purchase of tried on clothes on the retailer’s online site built into the mirror.
Categorizing automation improves UX and saves time
Manually categorizing products can be tedious and error-prone.
Computer vision can “see” products and automatically categorize them for inclusion in catalogs and properly warehouse them. Automated categorization is particularly vital in business segments that have continually changing product inventory types, like grocery stores.
It’s easy to see how these kinds of use cases greatly improve efficiency through computer vision automation.
Product attributes are important for accurate searches
It is common to find errors in product descriptions, particularly in large catalogs, which can negatively impact search and recommendation engines.
Computer vision can be used to correct existing product attribution mistakes, and to accurately discover missing visual product attributes, such as size or color, and add to product descriptions.
In this case study, we explore how machine learning can address attribution issues in e-commerce catalogs. In the example below, the shoe is misattributed as a flat.
As it stands now, users searching for sneakers would be unable to find this shoe. Also, users searching for “flats” would be presented this shoe in the search results, creating a distraction.
A deep learning algorithm flags the potential error as a computer-generated “second opinion”, so a human can then make a final decision on the potential correction.
Retailers with large catalogs need accurate attribution for successful search results. While visual search and visual product recommendations may find the product in spite of the misattribution, text searches will suffer.
Warehouse automation improves efficiency
There are many physical item movements inside a warehouse.
Computer vision and robotics can automate these tedious and tiresome tasks. Robots can detect, classify, and read the exact location of a particular item. The robotic arm can use computer vision to grab the piece precisely with an appropriate amount of force.
Amazon warehouses are excellent examples of computer vision, and artificial intelligence applied to make inventory movements faster, cheaper, and more efficient.
Inventory management controls cost
It is crucial to maintain accurate inventory management, as customer frustration runs high if they order items only to discover they are out of stock.
A reliable digital retail site requires accurate inventory that requires embedding availability information into the search index. Advanced computational techniques such as artificial intelligence and machine learning are used to maintain the accuracy of inventory counts.
Delivery innovations
Once a package goes out the back door for delivery, computer vision is still very much in use.
Computer vision, artificial intelligence, and deep learning complement robotics to aid in the physical movement of the package to reach its intended route, replacing much a significant amount of labor.
Conclusion
Computer vision is a discipline still growing and we have only shown some uses in production today.
Below are predictions of potential future use cases. As the technology matures, it is bound to become more ubiquitous, not only within online retail but in many more aspects of our daily lives.
-
Data-driven customization will pinpoint customer preferences
Algorithms based on individual user information such as social behavior, occupation, or preferences, will become increasingly common on e-commerce platforms. -
Humans will be back in the loop
Ironically, one of AI’s first uses was to remove humans from the loop. In the future, AI will re-integrate into the process of assisting humans, rather than replace them. -
“Search by image” will become predominate
Visual search take on a much more predominant role. The ubiquitous mobile phone camera makes “I want this” searches increasingly easier than text.
AL will graduate to designing, rather than just copying
Computer vision and artificial intelligence will advance the creative element into taking existing fashion cues and fabricate new ones. -
Product delivery will be revolutionized
Artificial intelligence, computer vision, and robotics today has only scratched the surface on the capabilities of package mobility. Delivery improvements hold much promise to improve the online retail industry for both customer satisfaction and corporate cost control.
Future posts will delve deeper into the solutions of AI in digital retail. We will cover the options available to companies looking to have their own computer vision experiences.
Subscribe to our blog to get the publishing update.