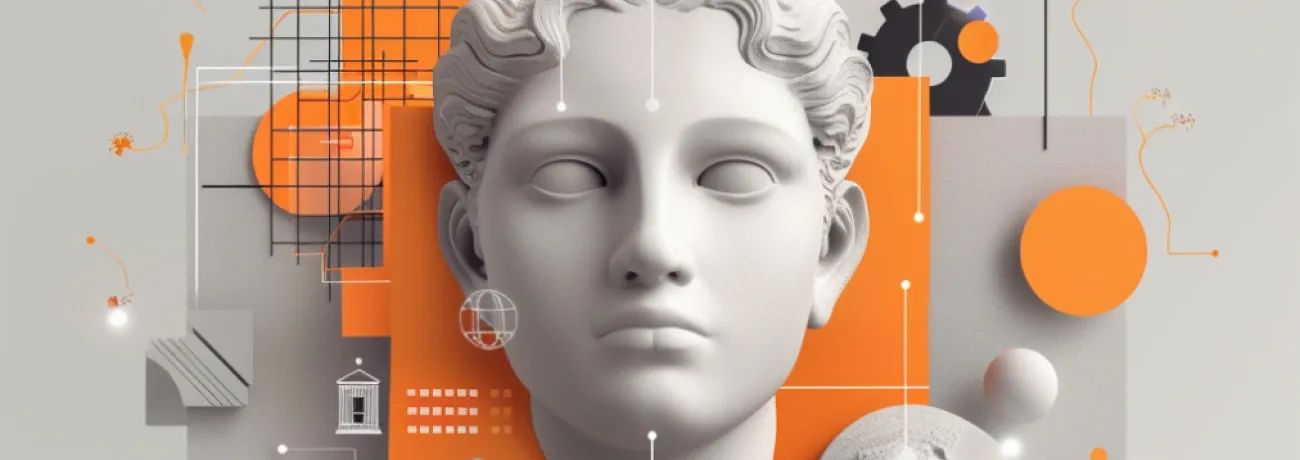
AI financial advisor: Assess investment suitability with GenAI
October 16, 2024 • 9 min read
- Suitability issues with alternative investments
- AI in wealth management
- Challenges of traditional investment suitability assessments
- Introducing the Grid Dynamics GenAI Investment Suitability Assistant
- Solution capabilities
- Under the hood | Agentic Retrieval-Augmented Generation (RAG)
- Comparing Naive RAG to Agentic Multimodal RAG
- Recap
- References:
AI is emerging as a transformative tool in regulatory compliance. Recent regulatory actions underscore the urgency of this technological shift.
Earlier in 2024, LPL Financial agreed to pay more than $6m in fines and restitution to settle claims brought by the Financial Industry Regulatory Authority (FINRA). The issue? Failure to adequately supervise transactions and recommendations made by its brokers, with FINRA citing concerns over 830,000 transactions and recommendations that didn’t meet regulatory suitability standards1.
This case is not an anomaly. A 2023 study by law firm Eversheds-Sutherland found that suitability violations comprise the majority of FINRA enforcement measures2.
In this blog post, we’ll explore how Grid Dynamics’ GenAI Investment Suitability Assistant Starter Kit offers a robust solution to investor and fund matching, alleviating some pressing regulatory challenges. We’ll cover the intricacies of current regulations, the unique difficulties posed by alternative investments, and how AI is poised to transform compliance processes in wealth management.
Suitability issues with alternative investments
Alternative investments, like Private Equity, provide the potential to leverage patient capital and generate alpha while also providing diversification benefits and access to a larger investment universe. However, this also comes with attendant risks related to illiquidity, capital calls, and extended lockup periods, which need to be communicated upfront.
This presents a distinct challenge to Financial Advisors and broker-dealers, particularly when determining suitability under the regulatory framework of FINRA Rule 2111 and Regulation Best Interest (Reg BI).
- FINRA Rule 2111 – Customer Suitability
FINRA Rule 2111 requires that broker-dealers, RIA, and Financial Advisors have a reasonable basis for recommending transactions and investment strategies. Notably, the FA has to account for the client’s financial situation and needs and specific factors like:
- Age
- Investment Objectives
- Risk Tolerance
- Financial Condition
- Diversification
An explicit regulatory mandate is thoroughly reviewing the investment features, including risks, rewards, and costs. Additionally, the advisor must understand the product’s market risks and how these risks could affect investors under various scenarios.
For traditional investments like stocks or bonds, these factors are easily assessed using standardized, easily digestible data. However, alternative investments can be vastly different. They involve intricate structures, high minimum investments, and illiquid assets, complicating suitability assessments. Advisors must sift through multiple legal documents, including PPMs, LPAs, and Side Letters, to fully understand the risks and terms of each investment.
In this context, manual processes become time-consuming and prone to error. Firms need a robust, scalable solution to automate much of the data extraction and analysis while ensuring each investment recommendation aligns with regulatory suitability requirements.
- Regulation Best Interest (Reg BI)
Set under Exchange Rule Act 15/-1(1)(2)(ii) and enforced by the SEC, this regulation sets the standard of care that fully applies to non-discretionary accounts and discretionary accounts under limited circumstances such as investment recommendations. It requires broker-dealers and others to exercise reasonable due diligence, care, and skill and have a reasonable basis to believe that a transaction or a series of transactions are in the customer’s best interest in light of the customer’s overall investment profile. Since 2021, over 30 firms and institutions have been hit with consent orders from FINRA for avoidable violations of Reg BI, with fines and restitution ranging from hundreds of thousands to millions of dollars.
- ‘40 Act Fiduciary Standard
The 1940 Investment Advisers Act establishes fiduciary duties for RIAs, refined through SEC interpretations and commissions. Landmark case laws that expand on the protections and obligations have included the following:
- SEC v Chenery Corp (‘43)
- SEC v Arleen W. Hughes (‘48)
- SEC v Capital Gains Research Bureau, Inc. (‘63)
- Transamerica Mortgage Advisors v Lewis (‘79)
- Share v Air Properties G. Inc. (2008)
- Robare Group v SEC (2019)
Public enforcement actions and consent orders, even for inadvertent violations, cause substantial reputational damage to the institution, which is then perceived as self-dealing at customers’ expense. This can take years to fix and is far more damaging.
This is why many wealth managers and RIAs turn to technology solutions to transform a manual and error-prone process.
AI in wealth management
According to a 2024 Mercer study, nearly 54% of investment advisors integrate AI into their operational processes, and 37% plan to use it in investment strategy or asset-class research. Other studies have noted that AI has reduced the time spent on manual data analysis at Wealth Management firms by over 12%. Investment management firms have reported a 7% lift in annual revenue from these solutions3.
This rising tide of AI adoption comprises use cases ranging from investment due diligence and management to compliance and customer service. GenAI, in particular, is playing a transformative role by enabling faster, more accurate data processing, personalized recommendations, and quicker decision-making.
Investment suitability is an integral part of the broader due diligence process, which includes evaluating investment products, market conditions, and compliance with the client’s Investment Policy Statement (IPS). AI, specifically GenAI, is uniquely positioned to streamline this process by analyzing vast datasets on the client’s financial profile and the investment products being considered.
Leveraging AI enhances the reasonable-basis suitability process by automating the analysis of vast datasets on investment products, market conditions, and regulatory requirements. It can rapidly extract critical insights from complex financial and legal documents, such as Private Placement Memos (PPM), Limited Partnership Agreements (LPA), and prospectuses, identifying potential risks, fees, and conflicts of interest. This enables advisors to understand the product’s characteristics better, helping determine whether it meets the reasonable-basis test for their clients.
By reducing manual effort and enhancing the depth of analysis, GenAI can enable investment advisors to fulfill their reasonable-basis suitability obligations more efficiently while offering recommendations that align with client goals and regulatory standards. This increases accuracy and speed and reduces the risk of human error while ensuring compliance.
The AI market in finance is expected to expand from 7.3 billion dollars in 2021 to 22.6 billion dollars by 2026, with the global AI market size projected to reach 1.85 trillion dollars by 20234. |
Challenges of traditional investment suitability assessments
Traditional investment suitability assessments face numerous challenges that hinder efficiency, compliance, and client satisfaction. Here are some of the critical issues:
Manual processes Fragmented communication channels lead to delays in gathering accurate client information. Operational inefficiencies in BO operations, including document management and data entry. Complex approval workflows create bottlenecks across departments. Poor system integration across FO, MO, and BO platforms. | Complexities of alternative investments Inefficient extraction and analysis of diverse document types. Complex multi-format data that hinders traditional automation from practical parsing. Aligning complex investments with investor profiles (risk tolerance, financial capacity, time horizon). Balancing portfolio impact through sector/ geo diversification and correlation. Risks related to long-term, illiquid alternative investments. | Regulatory compliance hurdles Complex regulatory demands around Reg BI and FINRA Rule 2111. Recommendations may fall short of best interest standards. Churning risk and conflicts of interest undermine trust. Inadequate documentation weakens audibility. |
Introducing the Grid Dynamics GenAI Investment Suitability Assistant
The GenAI Investment Suitability Starter Kit helps process and analyze multimodal data sources at scale. The core objective is to aggregate client suitability data from multiple internal and external sources, derive a semantic understanding of this information, and integrate it with a deep semantic analysis of investment documents (e.g., PE PPM/LPA) by leveraging multimodal LLMs for more informed decision-making.
This helps pose specific questions around portfolio fit, time horizon, liquidity, etc., that touch upon particular requirements around the reasonable basis standards. The kit can then be used to generate a fitment score, which provides the advisor with a sound basis for either accepting or rejecting the investment in the specific context of that particular client.
The GenAI Investment Suitability Assistant Starter Kit is a modern solution to enhance wealth managers’ decision-making by aligning investment options to investor profiles. It is designed to provide financial advisors with a comprehensive platform to navigate the complexities of investment recommendations. This ensures investment recommendations are tailored to individual risk tolerance, financial capacity, and long-term goals. Lastly, it streamlines the investment process, enhances compliance with regulatory standards such as Reg BI and FINRA Rule 2111, and provides a clear auditable decision trail.
Solution capabilities
Data integration and aggregation Ingests and aggregates data from diverse sources, including customer CRM, portfolio management tools, risk questionnaires, and third-party databases. Consolidates and structures client and investment data to enable seamless analysis. | Advanced analytics with LLMs Utilizes LLMs to extract and process critical information from complex financial documents, such as Private Placement memos (PPM) and limited apartment agreements (LPAs). Applies algorithmic techniques to understand entities and capture nuanced relationships between data points to generate meaningful insights. | Investor-fund matching Aligns private investment options to individual investor profiles, considering factors like risk tolerance, financial capacity, goals, and time horizons. Runs sophisticated similarity searches to identify best-fit investment opportunities for each client. |
Regulatory compliance Aids in meeting regulatory requirements regarding investor accreditation and suitability determinations. It helps demonstrate that recommendations are in the client’s best interest. | Suitability assessment and documentation Provides a quantitative, data-driven approach to evaluating investment suitability, balancing portfolio impact, and managing risks associated with alternative investments. Generates a transparent, auditable decision trail to document the suitability determination process. |
Under the hood | Agentic Retrieval-Augmented Generation (RAG)
The GenAI Investment Suitability Assistant Starter Kit’s technical foundation leverages advanced Agentic Retrieval-Augmented Generation (RAG) techniques. This differs from conventional RAG, which simply adds user inputs into the token window.
The idea of using agentic RAG is to provide a robust and efficient system for processing and analyzing multimodal data. This enables efficient and accurate information retrieval, personalized client management, and regulatory compliance.
Investment suitability assessment workflow
The RAG-powered investment suitability assessment workflow streamlines the process of analyzing client data and generating tailored recommendations. Here’s an overview of the key components:
- Data ingestion and vectorization
- Ingestion pipeline: The system begins by ingesting diverse data types, including documents and website content. These data sources are processed through a chunking and pre-processing library, broken down into manageable pieces, such as text, tables, images, and charts.
- Vectorization: Each chunk is then vectorized and converted into numerical representations using an embedded model. Comparing these vector representations enables the system to perform efficient searches and retrieval operations across large datasets.
- Machine Learning applications for data analysis
- Pipeline router: A machine learning-based pipeline router categorizes the chunks into different formats, such as text, tables, images, charts, and knowledge graphs (KG). This categorization ensures that each query can be matched with the most relevant data type.
- Query reformulation: When a query is submitted, machine learning models, including LLMs, are utilized to reformulate the query for optimal retrieval. This process may also incorporate memory from previous interactions to improve context and relevance.
- Quantifiable suitability scores generation
- Hybrid search: The system combines vector search and metadata search to retrieve relevant data chunks. These are then re-ranked by reference and chunk re-ranking algorithms, which factor in the specific context and constraints of the query.
- Answer agents: Leveraging the re-ranked data, the system’s answer agents generate responses, including quantifiable suitability scores. These scores are based on complex data analytics and tailored to specific financial profiles or investment strategies.
- Integration with existing systems
- Tools & LLM router: The technical architecture is designed to integrate seamlessly with existing CRM systems, portfolio management tools, and other financial software. This allows firms to incorporate suitability scores and analytics directly into their current workflows.
- Compute environment & tools: The system operates within a scalable compute environment, enabling integration with various tools and APIs to enhance functionality and data accessibility.
Comparing Naive RAG to Agentic Multimodal RAG
The evolution from naive Retrieval-Augmented Generation (RAG) to multimodal modern RAG has significantly enhanced the capabilities and performance of these systems. Here’s a comparison of their functionalities:
Functionality | Naive RAG | Multimodal Modern RAG |
Index-time processing | Pre-processing and chunking: Documents and websites are pre-processed, where content is chunked into manageable pieces (text, tables, images). Embedding model: These chunks are then processed through an embedding model that creates vectorized representations of the data, which are indexed for fast retrieval. | Chunking and pre-processing library: Advanced chunking methods are employed, tailored to handle diverse data types (text, tables, images, charts) with greater precision. Pipeline router: Data chunks are routed through specialized pipelines, allowing for better handling of multimodal content. The processed data is embedded with metadata and indexed for more effective search and retrieval. |
Query-time processing | Query reformulation: When a user initiates a query, the system reformulates the query to enhance context understanding, incorporating memory and domain-specific LLMs. Search and retrieval: The reformulated query searches the vectorized data, retrieving the most relevant embeddings to generate an answer. Answer generation: The system then generates responses based on the retrieved information, using LLMs to create a coherent and contextually appropriate output. | Query reformulation and contextualization: The query is reformulated with advanced context understanding, integrating long-term memory and domain-specific knowledge to ensure accuracy. Hybrid search: A hybrid search approach combines vector search and metadata search, significantly improving the relevance of retrieved data. Advanced reference re-ranking: Once data is retrieved, reference re-ranking and chunk re-ranking refine the results to ensure the most contextually appropriate information is presented. Citation and response generation: The system generates responses with semantic caching and guardrails to minimize errors like hallucinations, providing reliable citations and coherent answers. |
Recap
The fusion of advanced analytics, AI, and machine learning with traditional wealth management practices isn’t just an incremental improvement—it’s a shift that promises to change the way you serve your clients, offering new opportunities for personalization, efficiency, and compliance.
By leveraging advanced analytics and generative AI techniques, the starter kit enables wealth management firms to:
- Process and analyze large amounts of multimodal data efficiently
- Provide personalized investment recommendations that match clients’ financial goals and risk profiles
- Comply with regulatory standards like Reg BI and FINRA Rule 2111
- Improve workflows and boost advisor productivity
- Enhance client experiences through data-driven insights and customized strategies